Method
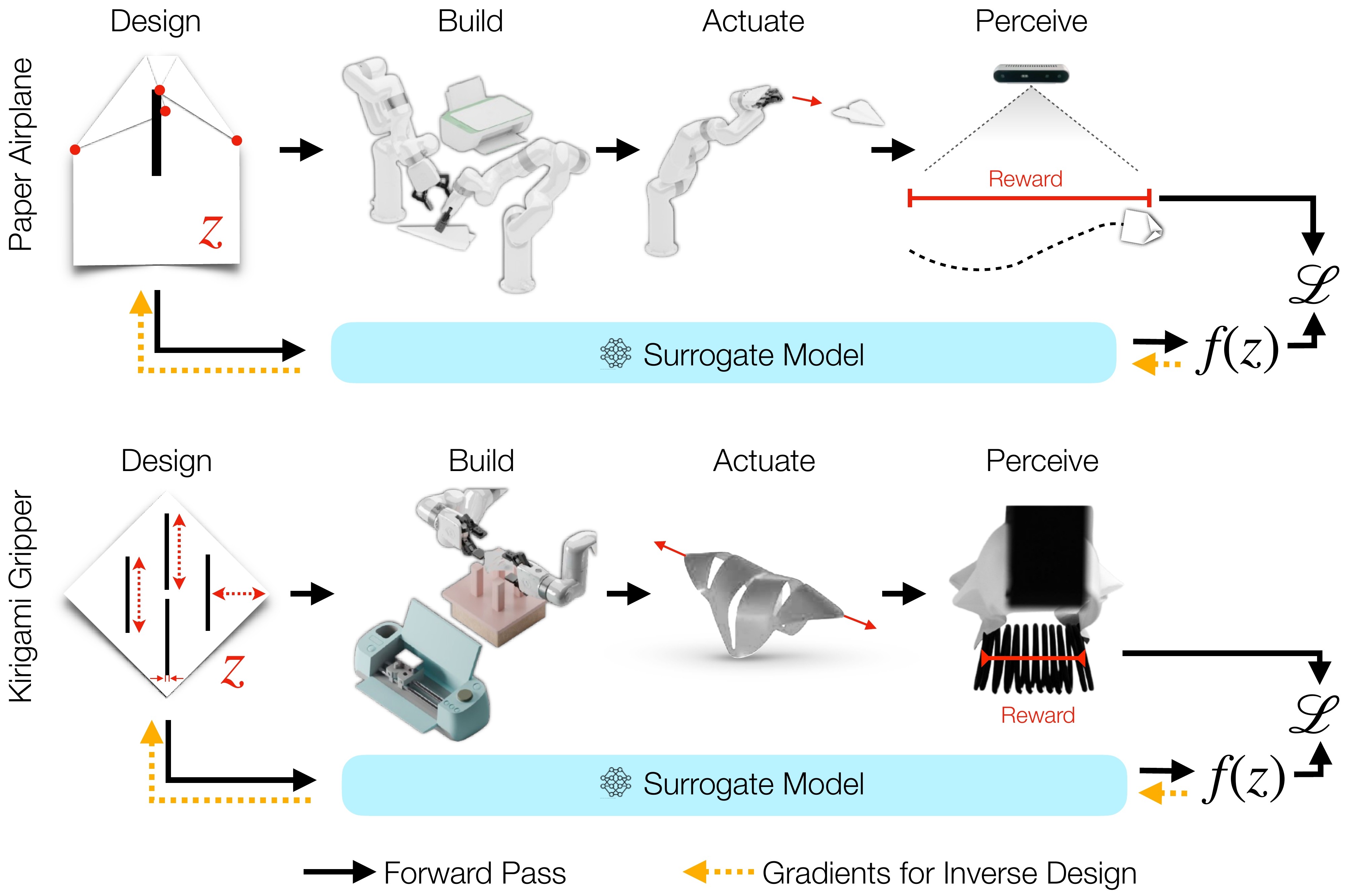
Paper is a cheap, recyclable, and clean material that is often used to make practical tools. Traditional tool design either relies on simulation or physical analysis, which is often inaccurate and time-consuming. In this paper, we propose PaperBot, an approach that directly learns to design and use a tool in the real world using paper without human intervention.
We demonstrated the effectiveness and efficiency of PaperBot on two tool design tasks: 1. learning to fold and throw paper airplanes for maximum travel distance 2. learning to cut paper into grippers that exert maximum gripping force. We present a self-supervised learning framework that learns to perform a sequence of folding, cutting, and dynamic manipulation actions in order to optimize the design and use of a tool. We deploy our system to a real-world two-arm robotic system to solve challenging design tasks that involve aerodynamics (paper airplane) and friction (paper gripper) that are impossible to simulate accurately.
Learning happens 100% in the real world without any simulation. Rendering is for visualization only.
@misc{liu2024paperbot,
title={PaperBot: Learning to Design Real-World Tools Using Paper},
author={Ruoshi Liu and Junbang Liang and Sruthi Sudhakar and Huy Ha and Cheng Chi and Shuran Song and Carl Vondrick},
year={2024},
eprint={2403.09566},
archivePrefix={arXiv},
primaryClass={cs.RO}
}